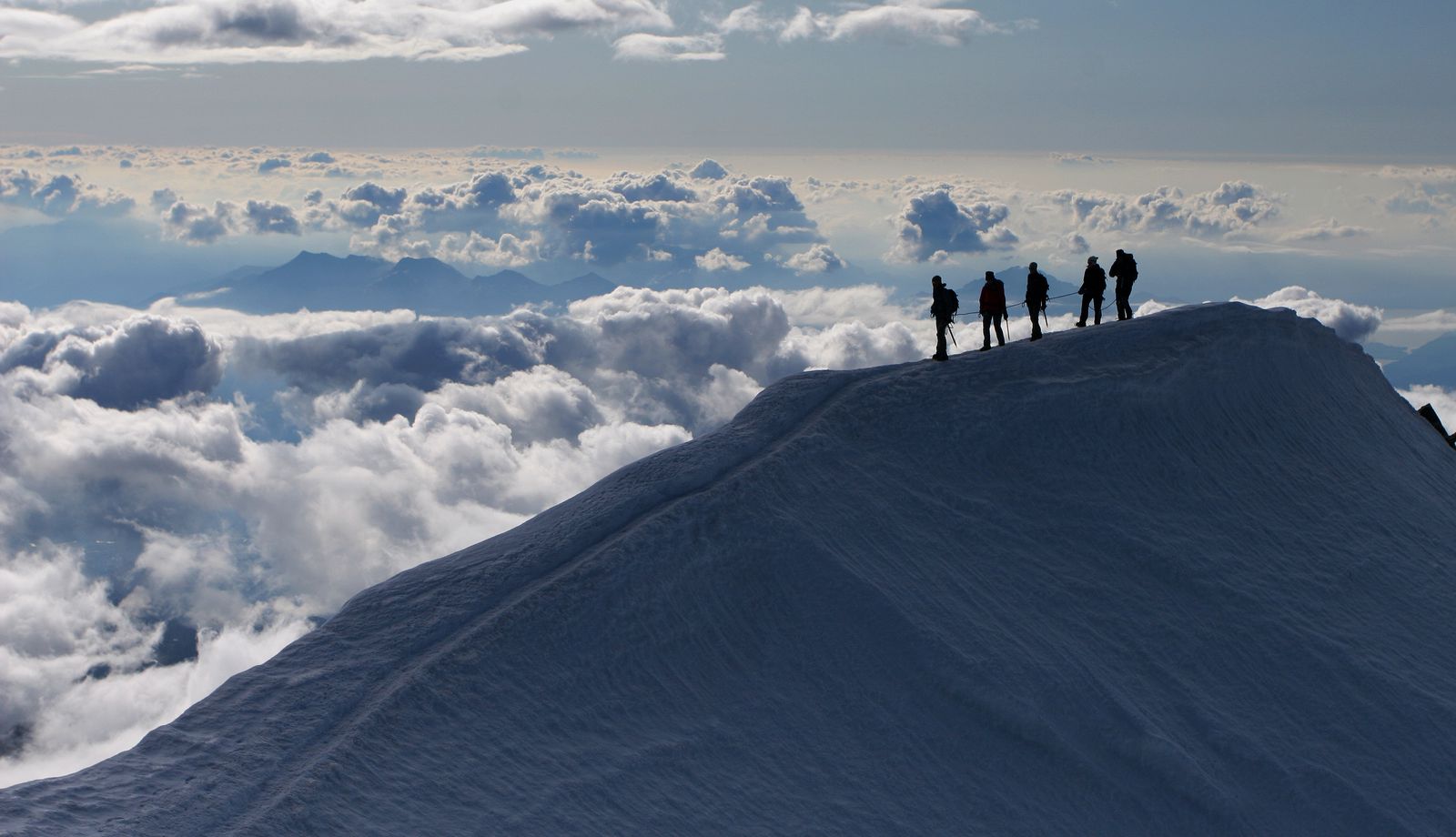
Excellence
Be in the leading pack with cutting-edge analytics solutions
Xcelerit is a leading provider of cutting edge analytics solutions for Quantitative Finance, Engineering, and Research. The company’s mission is to accelerate the pace of business, innovation, and scientific discovery.
Xcelerit’s extensive experience enables the firm to deliver full solutions from expert training, advisory, and bespoke products. Its distinct competitive advantage derives from the unique combination of domain specialist knowledge and cutting-edge software expertise. This allows the firm to forge the most efficient solutions to better address its clients’ needs.
Xcelerit is the maker of XAD, the fast and comprehensive C++ library for automatic differentiation. The company has received recognition as a finalist in the Red Herring Europe Top 100 award, the Red Herring Top 100 Global award, and the only two-time winner of HPCwire’s “Best use of High Performance Computing in Financial Services” award. Xcelerit satisfied customers include the leading firms in investment banking, asset management, and insurance. The firm informs and is informed through close partnerships with the world’s leading technology firms, including IBM, Intel, Nvidia, and many others.
Xcelerit help clients boost the performance of their compute-intensive software applications. The firm engagement model offers a unique combination of dedicated training, advisory, and custom software products. Clients can unlock massive IT infrastructure costs savings, reduce their carbon footprint to meet their green agenda, and leverage new application and volumes that weren’t possible before.
Xcelerit help clients modernise large legacy code bases developed over decades. It allows clients to migrate from outdated technologies, implement cutting-edge software development practices, and be positioned at the frontline of technology innovation. The firm engages with clients via a unique combination of dedicated training, advisory, and custom software tools.
Xcelerit believes in open source software and is committed to support the open source software community.
Xcelerit is the maintainer of XAD, the fast and comprehensive C++ library for automatic differentiation. It targets production-quality code at any scale, striving for both ease of use and high performance.
Since its founding in 2010, Xcelerit has been cultivating a culture of excellence and innovation.
We are always on the lookout for exceptional people of all experience and levels in the following areas: Software Engineering, Quantitative Finance, DevOps, and Sales. As an integral member of our team, you will be actively working on all aspects of our technology and services. Working closely with other team members and founders, you will create pragmatic solutions to the business challenges facing our clients.
If you are interested in helping businesses create a virtuous cycle of technical innovation and commercial success, enjoy problem solving and engineering challenges, and believe in elegant software, talk to us! We provide excellent benefits and competitive pay. You can reach us via email careers(at)xcelerit(dot)com or check our current job openings on LinkedIn.
27 Upper Pembroke Street
Dublin 2
D02 X361
Ireland
+353 1 254 2160
Please give us some details so we can answer your request.